Introduction
Along with the economic improvement and a rapidly changing society, modern people gradually have showed more attention to the functional health such as anti-aging, and the prevention of disease (Choi et al., 2003). Therefore, it is predicted that the demand for functional products to maintain good health will increase. The tea, one of the major favorite foods, has been getting more attention due to not only the simple thirst-quenching role, but also the pharmaceutical efficacies such as the antioxidant, the anti-cancer or the immune enhancement effects activated by the phytochemicals. Je (2015) reported that the important physiological activities of the plant compounds are important anti-inflammatory, anti-hypertensive, and anti-oxidant physiological effects. Recently, the increased interest of the natural substances as an alternative medicine and the market expansion for the tea drinks using health functional extracts have been reported in many researches (Cho et al., 2010; Lee, 2011: Mun et al, 2007; Park et al., 2000).
In a situation where tea can be noticed, in order for tea-related researches to be used as the useful resource for the future tea drink research and development, it is necessary to classify the plants, and the phytochemical ingredients and pharmaceutical efficacy of the plants. Such systemic classification and organization of the data will be used for future research as the sources and the knowledge for the tea research.
Social network analysis has been used as a way to build a knowledge system for the subject and keywords of recent studies. Social network analysis can make it possible to macroscopically understand the research trends in a particular field with a variety of perspectives. In particular, if the research data is large, it is possible to analyze the relationship and attribute related to the individual perspective not well identified by the traditional approaches (Kwahk, 2017).
In the research for determining the relationship between the raw material and its efficacy as shown in this study, the research, and for identifying the plant, chemical composition and the hierarchy of its efficacy, the analysis with a measurement method capable of comparing the positional advantage and effect in the overall research trend is needed (Kim and Kim, 2016). Social network analysis has been widely utilized in various fields such as the tourism field (Chung et al., 2019), the restaurant menu (Kong and Yu, 2014), the education (Heo, 2016b), the sports psychology (Park et al., 2016) and new product recommendations (Cho and Bang, 2009). Social network analysis using the keywords related to the business administration research made it possible to confirm the main research theme of future business administration based on the appearance frequency analysis, the centrality analysis, the aggregation group analysis and the awareness analysis, and to understand the process of changing knowledge structure in business administration research (Kim et al., 2015).
Thus, we investigated the plant compounds as the easy-drinking tea and their pharmaceutical efficacies. However, the classification of the tea is so vast that it is difficult for non-professional people to figure out the results. So we analyzed more than 50 domestic scientific articles and classified them into seven major categories based on the keyword “tea drink”; 1. Advertising marketing with the brands (3 scientific articles), 2. Quality-related investigation (5 scientific articles), 3. New products and preference market trends (11 scientific articles), 4. Tea drinks and health-related functionality (19 scientific articles), 5. Analysis of the compounds (3 scientific articles), 6. Designs (7 scientific articles), and 7. Manufacturing processes (3 scientific articles). Among the categories above, tea drinks and health-related functionality were the most studied topics followed by new products and preference, market trends and then designs. Surprisingly, the study of ingredient analysis and manufacturing processes was relatively fewer (data not shown). Researches on tea drinks are mainly related to the chemical properties, the functional components and the anti-oxidants (Ahn et al., 2012; Kang et al., 2009; Ko et al., 2006), and safety and activity tests for the heavy metal toxicity by using the in vivo animal experiments (Choi et al., 1994; Ji and Ha, 2012). However, there still need more studies in terms of quantity compared to the researches related to the humanities and social issues.
Therefore, this study suggests a knowledge map as an appropriate methodology to provide an explanation of the relationship between the raw material plants and the phytochemical ingredients and pharmaceutical efficacy of the tea drink in situations in which tea is likely to draw attention. This methodology is a tool for applying social networks analysis, a technique in data analytics. In this study, knowledge maps context the relationship between raw materials plants and the phytochemical ingredients and the relationship between the chemical ingredients and pharmaceutical efficacy. Also, the hierarchical matching of two knowledge maps enables the matching of integrated information. Through this process, we intend to infer the relationship between raw materials plants and pharmaceutical efficacy and provide objective information based on data for the development or research of tea drink products. And we want to provide insightful information in identifying research trends in plant-related fields for the food industry.
Materials and methods
In order to collect the data on the tea drinks, three experts in the field of plants and food participated in this study for searching scientific articles. Experts found out the names of the plants from the tea drinks and then searched the scientific articles by using these plant names as the key words.
The scientific articles containing the selected key words in the abstracts and the body of the scientific articles were collected. The scientific articles were collected without the year limitation because raw material plant names and their pharmaceutical efficacy were not affected by the year of paper publication. Based on these criteria, 892 scientific articles were selected.
When the published year of the scientific articles was compared, there were 7 scientific articles before the year 1990, 30 scientific articles from the year 1991 to the year 2000, 245 scientific articles from the year 2001 to the year 2010, and 610 scientific articles from the year 2011 to the year 2019, respectively. About or the 96% of all selected scientific articles was after the year 2000.
As the most mentioned names of the tea plants, there were the Camellia sinensis, the citrus, the Panax ginseng, the pomegranate, the chamomile, the strawberry, the blueberry, the dandelion, the lemonbalm and the ginger in many order.
The data collected in this study was compiled in the form of analyzabel data. The data is reconstructed through clustering and data transformation. Once the data is available for analysis, the social network analysis proceeds.
The types of the raw material plants, the chemical ingredients, and the pharmaceutical efficacy sorted from the 890 scientific articles were 232, 1114 and 275, respectively. The clustering process was carried out to increase the usefulness of the data because there were the numbers of the raw plants, chemical ingredients and pharmaceutical efficacy that were broken down into one or two.
Through clustering, the structure of the data has been reclassified to reflect specific phenomena more clearly. This course was conducted by expert evaluation. Three experts related to plants and food were reclassified and categorized according to the similarity of contents of the raw material plants, the phytochemical ingredients and pharmaceutical efficacy. Clustering focused on targets with one or two frequencies. The reclassified data through clustering consisted of 115 raw material plants, 540 phytochemical ingredients and 116 pharmaceutical efficacy.
After clustering names of the categories, conversion of the data was performed to build a knowledge map of the names of the raw material plants and their pharmaceutical efficacy. To match the name of the raw material plant to the pharmaceutical efficacy, the data were organized based on the phytochemical ingredients related to the raw material plants and the pharmaceutical efficacy. For this purpose, the selected scientific articles were classified into two groups; a group of the raw material plants and their phytochemical ingredients, and a group of the phytochemical ingredients and their pharmaceutical efficacy (Table 1 and Table 2).
Plants | Chemical | ||||
---|---|---|---|---|---|
Acaricide | Acetaldehyde | Acetaminophen | Acetic acid | ⋯ | |
Acai berry | ⋯ | ||||
Adzuki bean | ⋯ | ||||
Angelica | ⋯ | ||||
Apple | 1 | ⋯ | |||
⋮ | ⋮ | ⋮ | ⋮ | ⋮ |
The blank is zero (data not shown).
Chemical | Efficacy | ||||
---|---|---|---|---|---|
DNA demage relief | Liver function improvement | Tonic effect | Menopause symptom relief | ⋯ | |
Acaricide | ⋯ | ||||
Acetaldehyde | ⋯ | ||||
Acetaminophen | 1 | ⋯ | |||
Acetic acid | ⋯ | ||||
⋮ | ⋮ | ⋮ | ⋮ | ⋮ |
The blank is zero (data not shown).
According to the Kwahk (2017), the data distinguished from each other by the rows and columns were named as the 2-mode, and two measured objects belonged to two different social entities. The association between the social entities corresponding to the row and the column was converted into a vector value distinguished by the cell value of the matrix and the binary number of 0 and 1. If two social entities were associated or not associated with each other, the vector value was described as the 0 and the 1, respectively. As described in Kwahk (2017), the rows and columns will be described as the actor and the event for technological convenience, respectively. The 2-mode matrix in this study is an asymmetric matrix with different lengths of rows and columns.
There are two 2-mode data matrices in this study. One is a data matrix in which the action is a raw material plant and the event is a phytochemical ingredient, as shown in Table 1. The other is a data matrix in which the action is a phytochemical ingredient and the event is the pharmaceutical efficacy, as shown in Table 2. The blank in the table means the case where it has not been studied in the collected scientific articles.
To build a knowledge map for the raw material plants, the phytochemical ingredients and the pharmaceutical efficacy, social network analysis was carried out in the data that were converted into the two-mode matrix. The social network analysis was performed by the UCINET1 program (Borgatti et al., 2002) that can analyze a key concept representing the characteristics of the network and that is able to utilize the various network analysis techniques (Kim and Kim, 2016). In this study, the relationship of the networks was analyzed by using the 2-mode network graph applying graph theory layout.
This study utilized the density, degree of centrality, closeness centrality, betweenness centrality among the central concepts of social network analysis. A mathematical description of each concept is shown below.
The node called a node or a vertex (Butts, 2008). Each element of a row and column placed in a data matrix, such as the above-mentioned actor or event (Denny, 2014). In this study, all raw material plants and phytochemical compounds and pharmacological potency collected correspond to nodes. And connections or relationships between nodes are called links (Butts, 2008).
The density was examined to confirm the cohesion of the network as follows below.
The density refers to the number of the links that are maximally connected to the 2-mode network consisted of the raw material plants and the phytochemical ingredients (de Laat et al., 2007). The density D is the value that the number of links L in the network is divided by the product of the number of nodes n1 in the raw material plants and the number of nodes n2 in the phytochemical ingredient.
Relationship of the connections can be shown not only from the entire network perspective, but also from the perspective of individual nodes.
The Centrality focuses on each node in the network. Measures how much nodes interact with other nodes in the network. The more nodes connect to other nodes in the network, the greater the Centrality becomes (de Laat et al., 2007). Centrality is an indicator of the connection, and 10 objects with high centrality were summarized (Table 3). From a node perspective, the connection shows the first connection centrality. Kwahk (2017) suggested that the Degree of Centrality of 2-mode network is defined as the number of relationship connected to other social entities and standardized to the size of the network. The expression of the connection centrality of the actor is as follows.
Table 4 is arranged in descending order according to the centrality in a two-mode network consisting of the raw material plants and the chemical ingredients. The three values were bolded in the order in which the centrality was highest.
C′D(Ni) of the actor is the standardized value of the connection centrality CD (Ni) of the node Ni and the n2 is the number of nodes in the event mode set A. In addition, connection centrality of the event has the same structure as the connection centrality of the actor (e.g. the raw material plant) does.
In the graph, the Closing Centrality is a measure of the Centrality in the network, calculated as the reciprocal of the shortest path length sum between the nodes and all other nodes in the graph (Bavelas, 1950). Thus, the more nodes are in the center, the closer they are to all other nodes. The Closeness Centrality is expressed as a reciprocal of the sum of the distance between all other nodes in the network (Sabidussi, 1966). The closeness centrality of the actor is as follows.
The C′C(Ni) of the actor(e.g. the raw material plant) is the standardized closeness centrality of the node Ni, and CC(Ni) as the sum of the shortest distance is the closeness centrality of the Ni. The n2+(2n1 − 1) is connected to all nodes with the shortest distance 1 in the 2-mode network, and to the other nodes belonging to the same node with the shortest distance 2. The closeness centrality of the event is also expressed in the same structure as that of the actor.
To confirm the importance of a particular node, this study compared the degree of passage through a particular node. Betweenness Centrality represent the ratio of the path containing a node in the shortest path of all node pairs (Anthonisse, 1971; Freeman, 1977). The equation on the Betweenness Centrality of the actor is as follows.
s=quotient of (n1−1)/n2
t=remainder of (n1−1)/n2
The standardized betweenness centrality C′B(Ni) of the node Ni is the maximum betweenness centrality CB (Ni) of the actor(e.g. the raw material plant) mode A divided by the value of the betweenness centrality C′B(A*) of the actor(e.g. the raw material plant) i.
Results
The frequency analysis were to the raw material plants, phytochemical ingredients and pharmaceutical efficacy of the tea drinks. Twenty names were selected from the scientific articles based on the frequency of raw material plants, phytochemical ingredients and pharmaceutical efficacy. As three major names of the raw material plants, tea (C. sinensis) with 373 cases, citrus with 248 cases and dandelion with 137 cases were 11%, 7.9% and 4.4%, respectively. In the names of phytochemical ingredients, the frequency rates of phenol with 392 cases, flavonoid with 249 cases and Catechin with 108 cases were 12.5%, 7.9% and 3.4%, respectively. In the names of pharmaceutical efficacy, the frequency rates of the anti-oxidants with 1960 cases, anti-inflammatory with 771 cases and anti-cancer with 685 cases were 28.3%, 10.3% and 9.9%, respectively.
The 2-mode data between the raw material plants and phytochemical ingredients were expressed in a 2-mode network graph (Fig. 1). The raw material plants and their phytochemical ingredients were expressed as a circles and a square. The raw material plants and their The phytochemical ingredients was applied to the directional link. The raw material plants and their The phytochemical ingredients was applied to the directional link. In this study there are large number of objects, and the number of links was limited to make the graph visually clear.
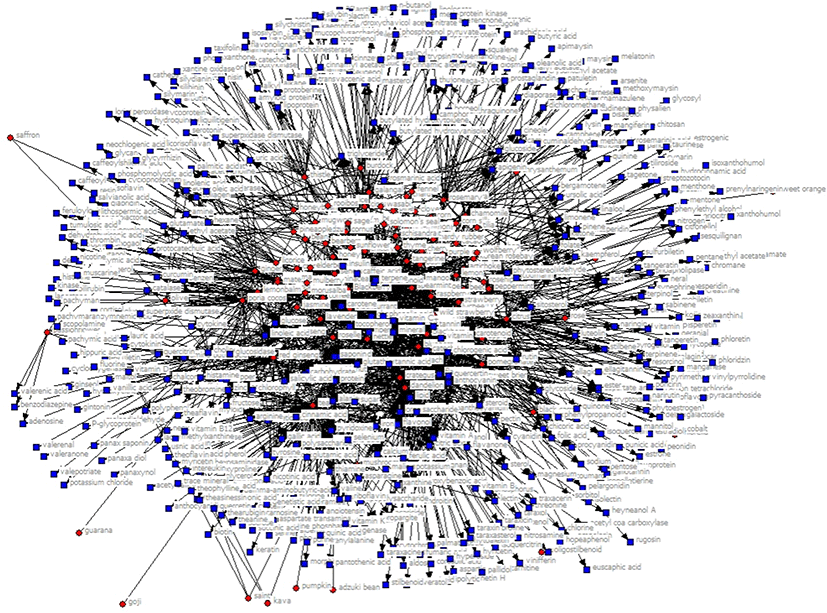
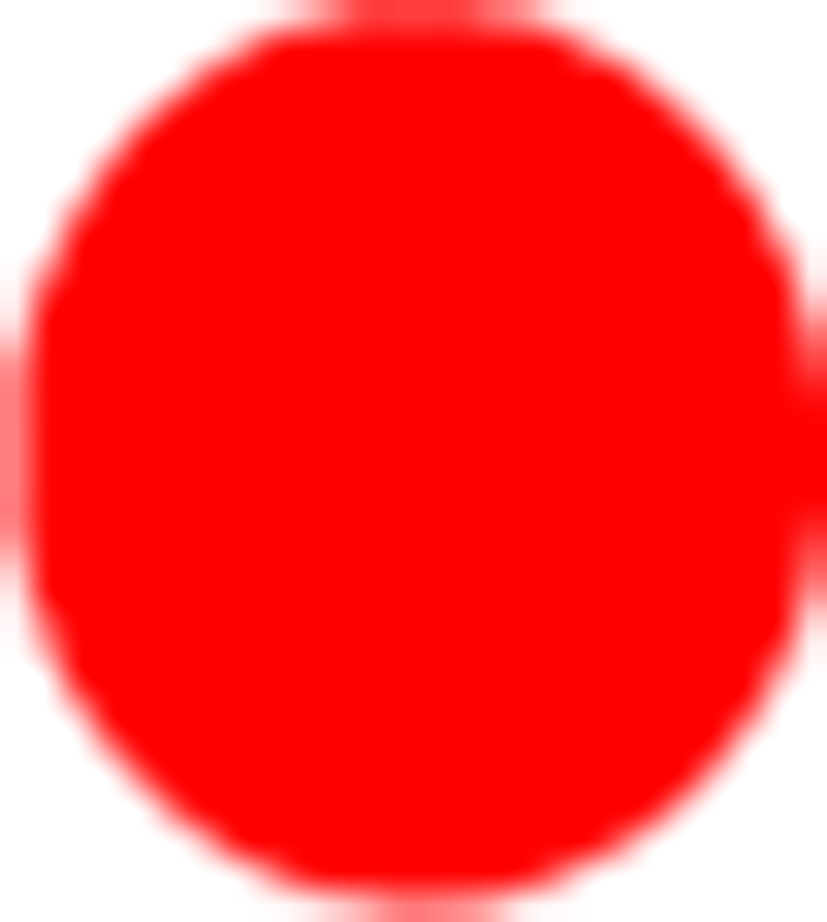
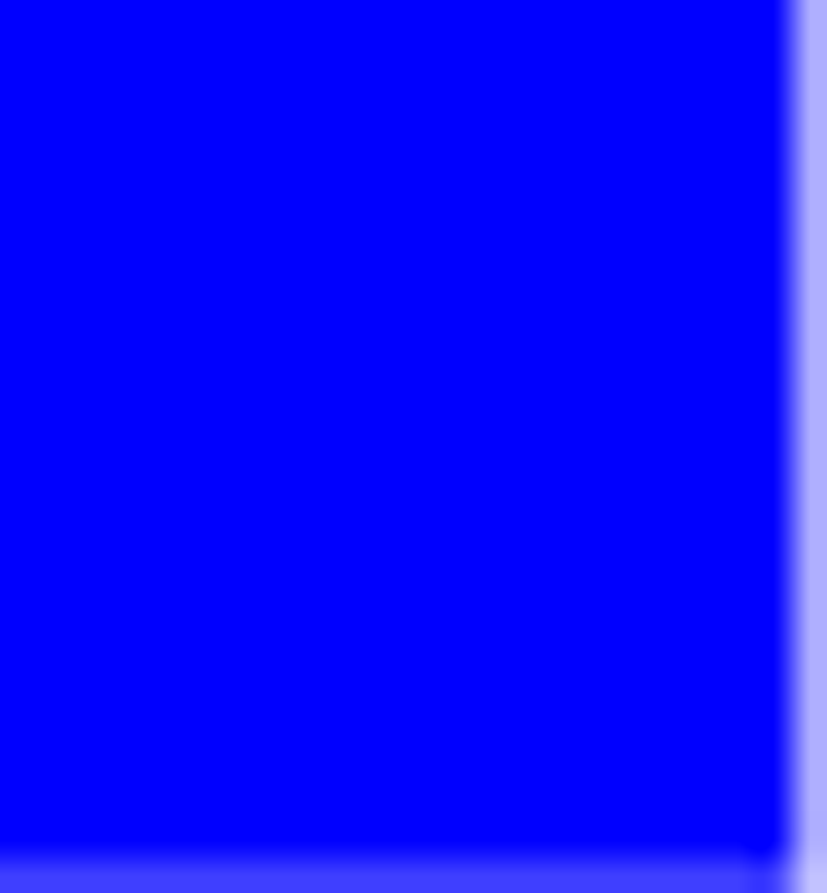
As you can see in Fig. 1, the network graph of the raw material plants and the phytochemical ingredients are a single circular structure. In the analysis of social networks about general articles, nodes that deviated from the central circle are found (Heo, 2016a), but in this study, no deviated nodes were found. So, it can be seen that the most raw material plants and phytochemical ingredients were used redundantly in many studies.
In Fig. 1, the tea (C. sinensis), the citrus and the dandelion with high frequency would be likely located near the center according to the graph mechanism of the social network. The phytochemical ingredients showing high frequency of connections to the raw material plants were the phenol which was located near the center of the network by the mechanism of the graph and of which the connected link was most dense.
The density of the 2-mode network between the raw material plants and the phytochemical ingredients was 0.029. The results show that the density of the link connected to the node corresponding to the raw material plants and phytochemical ingredients was not high. The density of the link by the bidirectional network graph seemed to appear dense due to a large number of categories for raw material plants and phytochemical ingredients. However, the results suggest that it would still need the further studies on the various raw material plants and their phytochemical ingredients.
Three names of the raw material plants with the high connection centrality were the C. sinensis, the hop and the citrus, and names of phytochemical ingredients with the high connection centrality are the phenol, the flavonoid and the vitamin C in many order (Table 3), respectively. These data indicate that the raw material plants and the phytochemical ingredients with the highest research frequency were the C. sinensis and the phenol, respectively, and have the greatest influence in tea drink research.
The raw material plants and the phytochemical ingredients with the high closeness centrality were the red chicory, the tea (C. sinensis), the citrus, the aglycone, the phenolic acid and the phenol, respectively. The raw material plants and phytochemical ingredients with high closeness centrality play a role in the connection as a hub does.
The results show that the raw material plants with high betweenness centrality are thetea (C. sinensis), the citrus and the pomegranate, and that the phytochemical ingredients with high betweenness centrality are the phenol, the flavonoid and the vitamin C, respectively, suggesting that the raw material plants with high betweenness centrality have been mentioned as various phytochemical ingredients in tea drink related studies and that the phytochemical ingredients with high betweenness centrality have been relatively more studied in other raw material plants compared to other phytochemical ingredients with low betweenness centrality.
Among major three raw material plants showing high connection, closeness and betweenness centralities between the raw material plants and the phytochemical ingredients, tea (C. sinensis) and citrus as the raw material plants and the phenol as the phytochemical ingredient were observed in common, respectively. From the points of view of connection and closeness centralities, we identified that the tea (C. sinensis)and citrus as the raw material plants and the phenol as a phytochemical ingredient have a positional advantage in the study of tea drinks. Also, our data suggest that tea (C. sinensis) and citrus as the raw material plants and the phenol as a phytochemical ingredient have been actively studied as the major subjects of the researches. According to the betweenness centrality, the raw material plants C. sinensisand citrus were most likely to have the same ingredients as the those of other raw material plants, and the phytochemical ingredient phenol can be considered as the most actively studied chemical ingredient of the raw material plants.
The 2-mode data between the phytochemical ingredients and their pharmaceutical efficacy were obtained by using a 2-mode graph (Fig. 2). To distinguish between the two elements, such as Fig. 1, the phytochemical ingredients were set as circles and their physical effects were set as squares. For the reasons shown in Fig. 1, the graphs of phytochemical ingredients and their physical efficacy also have a limit on the number of links. Also, the number of links was set to more than one case because the graph is focused on determining the distribution of the data as the form of the distribution.
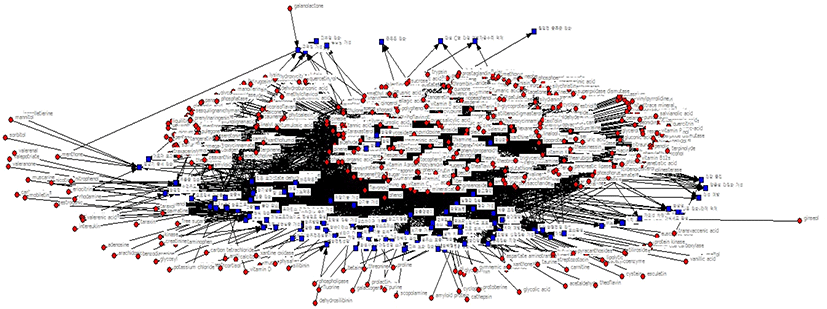
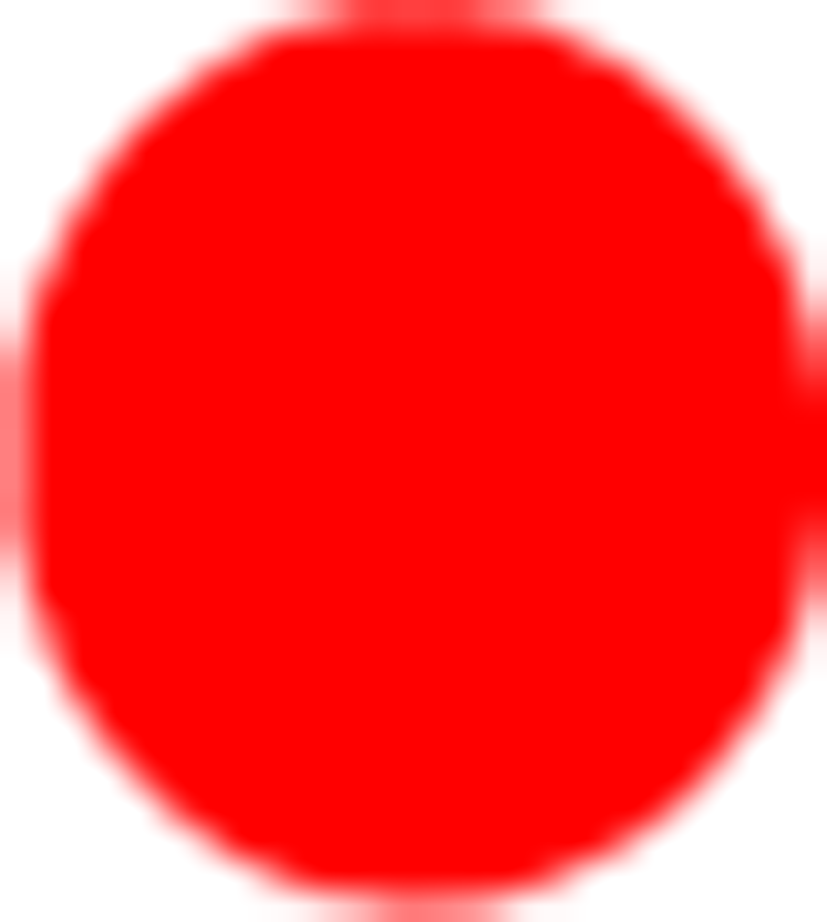
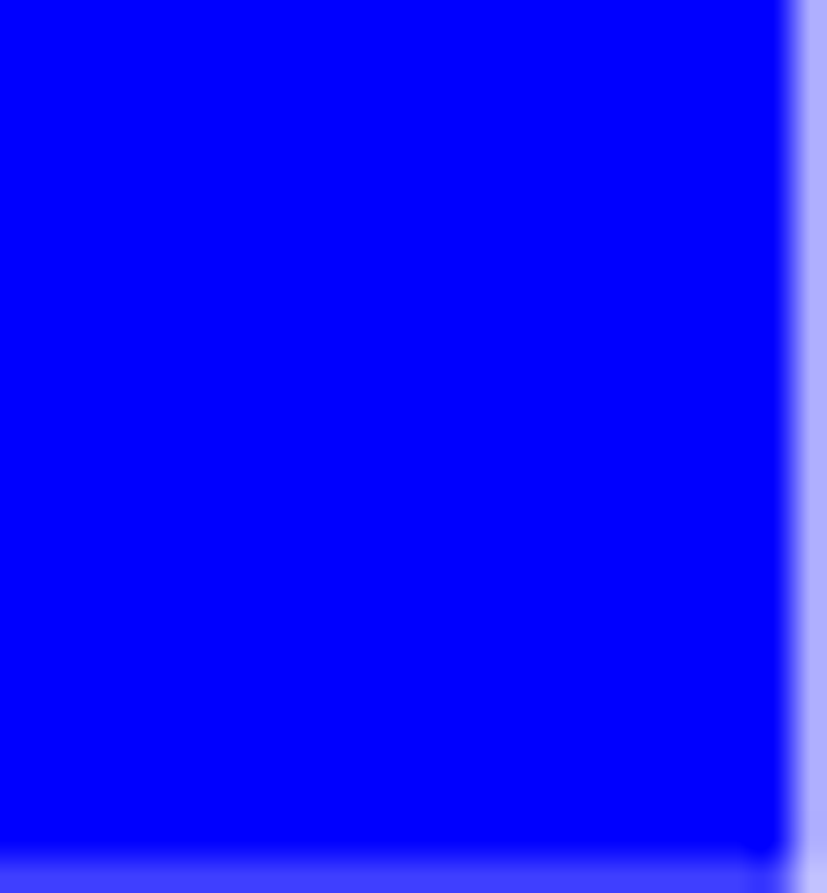
According to the analysis results, there was no isolated network in the shape of the graph when the number of links was more than one. The most important phytochemical ingredients were central, and the pharmaceutical efficacy was in the periphery. Fig. 1 was a circular model while Fig. 2 was an oval shape. The centrality of phytochemical ingredients and the neighboring pharmaceutical efficacy was not uniform, unlike those of raw material plants and phytochemical ingredients.
In the analysis results, the density of the association between the phytochemical ingredients and their physical effectiveness was higher than that between the original material plants and the phytochemical ingredients. In relationship between phytochemical ingredients and their pharmaceutical efficacies, connection density with the 0.45 showing the cohesion of the network was also higher than that between the raw material plants and their phytochemical ingredients. This observation suggests that the matching between phytochemical ingredients and their pharmaceutical efficacy is more active than that between raw material plants and their phytochemical ingredients.
The centrality of the 2-mode network between the phytochemical ingredients and their pharmaceutical efficacy was summarized only 10 objects with a high centrality as shown in the Table 4. The phytochemical ingredients and their pharmaceutical efficacy with high connection centrality were the phenol, the flavonoid and the mineral in the raw material plants, and the anti-oxidant, the anti-inflammatory and anti-microbial functions in the pharmaceutical efficacy, respectively. The phytochemical ingredients and their pharmaceutical efficacy with third rank closeness centrality were the phenol, the flavonoid and the mineral in the chemical ingredients and the anti-oxidant, anti-inflammatory and anti-microbial functions in the pharmaceutical efficacy, respectively. The phytochemical ingredients and their pharmaceutical efficacy with third rank betweenness centrality were the phenol, the flavonoid and the catechin in the phytochemical ingredients, and the anti-oxidant, anti-inflammatory and anti-microbial effects in the pharmaceutical efficacy, respectively. Specially, the phenol and flavonoid among the phytochemical ingredients were mostly connected to the pharmaceutical efficacy, and then showed the highest degree of the connection with the various pharmaceutical efficacy.
Table 4 is organized in descending order according to the centrality in a 2-mode network consisting of chemical ingredient and pharmaceutical efficacy. The three values were bolded in the order in which the centrality was highest.
Pharmaceutical efficacy including anti-oxidant, anti-inflammatory and antimicrobial functions also showed high degree of connection to the connection, closeness and betweenness centralities. In this study, the anti-oxidant was the most frequently mentioned as the pharmaceutical efficacy of the various phytochemical ingredients.
Among connection, closeness and betweenness centralities between phytochemical ingredients and their efficacies, the phenol was one of three phytochemical ingredients showing the highest centrality. Also, anti-oxidant, anti-inflammatory and anti-microbial functions were most common three pharmaceutical efficacy. From the centrality point of view, the phenol is most actively associated with the pharmaceutical efficacy and is likely to share the same pharmaceutical efficacy with other phytochemical ingredients. The anti-oxidant, anti-inflammatory and anti-microbial functions as the leading pharmaceutical efficacies have been seen most actively. Specially, the flavonoid is a major phytochemical ingredient in the field of tea research because it is connected to the various phytochemical ingredients and pharmaceutical efficacy.
Discussion
This study identified the characteristics of the relationship between keywords in tea drink-related research through social network analysis. Unlike general keyword analysis (Heo, 2016a), the social network analysis was conducted hierarchically on the matching between the raw material plants and their phytochemical ingredients and between the phytochemical ingredients and the pharmaceutical efficacy. Hierarchical analysis was conducted in two procedures: the network between the raw material plants and their phytochemical ingredients and their pharmaceutical efficacy.
The density of the 2-mode network was not high in the matching results through network analysis between the raw material plants and their chemical ingredients. This means that studies on the raw material plants and their phytochemical ingredients are biased. In particular, tea (C. sinensis) and citrus among the raw material plants and phenol, flavonoid among the phytochemical ingredients belong to them. This biased research trend can also be seen in the connection center, proximity center, and mediated center between the raw material plants and the phytochemical ingredients. tea (C. sinensis) and citrus, phenol and flavonoid can see that they can have multiple connection opportunities with the raw material plants or the phytochemical ingredients (Heo, 2016a; Jung and son, 2015). Thus, tea (C. sienesis) and citrus, phenol and flavonoid can be seen as the keywords for the most notable research in tea drink-related studies. From the point of view of the mediated center, tea (C. sinensis) and citrus were most likely to have the same phytochemical ingredients as the other raw material plants.
The density between the phytochemical ingredients and the efficacy of pharmaceuticals was higher than that of raw materials and the phytochemical ingredients. This can be said to be more active in the study of tea drinks than in matching the phytochemical ingredients according to the raw materials. Results showed that the raw material plants with high frequency were the tea (C. sinensis) and the citrus, and the pharmaceutical efficacy with high frequency were the anti-oxidant, anti-inflammatory and anti-microbial functions in this study of tea drinks, respectively.
In tea drink research, the tea (C. sinensis) and the citrus as the raw material plants and the phenol as a phytochemical ingredient are the major research topics with the most attentions (Zhao et al., 2019). The anti-oxidant, anti-inflammatory and anti-microbial functions have most frequently appeared as a mediator between the raw material plants and the phytochemical ingredients, and been considered as the key pharmaceutical efficacy of the phytochemical ingredients in the tea research (Bhatt et al., 2010; Mahmood et al., 2010; Namita et al., 2012). The tendency of high centrality in the raw material plants and the pharmaceutical efficacy may be likely due to the consequence of the social interest. Recently, the research using the social network analysis has increased as seen in widely used market analysis cases (Hwang et al., 2019). In the future studies, it would be necessary to directly match the pharmaceutical efficacy to the raw material plants based on the knowledge maps, and then understand and standardize the relationship of the pharmaceutical efficacy to the raw material plants.
As shown in earlier researches recommending the new products based on the social network analysis (Cho and Bang, 2009), the knowledge maps of this study on the raw material plants and the pharmaceutical efficacy would give new insight into the tea drink researches and industry. Most of the 892 scientific articles used in this study were based on in vitroor animal in vivoexperiments rather than direct experiments on humans. This study, which applied social network analysis corresponding to data analytucs, provides information on trends in tea beverage-related research from a macroscopic perspective, as in other keyword analysis articles (Heo, 2016a; Jung and son, 2015) on research subjects. It also provides the quantitative information into enabled and somewhat disabled topics. This information will provide a methodological perspective on how active research can be conducted between various topics by allowing the research characteristics of the subject to be identified in the course of research and development on tea drinks.